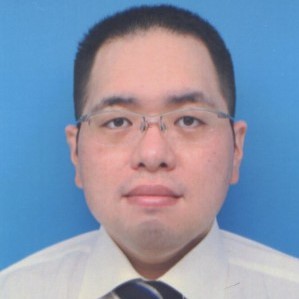
五十嵐 岳教授
Gaku Igarashi
- 研究分野
- 統計学
- 担当科目
- 統計学特論I、統計学演習
略歴
2010年:北海道大学経済学部経済学科 卒業
2012年:北海道大学大学院経済学研究科現代経済経営専攻修士課程 修了
2015年:北海道大学大学院経済学研究科現代経済経営専攻博士後期課程 修了 博士(経済学)取得
2015年:筑波大学システム情報系社会工学域 助教
2021年:学習院大学経済学部 准教授
主要業績
“Nonparametric direct density ratio estimation using beta kernel”, Statistics, Vol.54, Issue 2, pp.257-280, 2020.
“Multiplicative bias correction for asymmetric kernel density estimators revisited” (with Kakizawa, Y.), Computational Statistics and Data Analysis, Vol.141, pp.40-61, 2020.
“Limiting bias-reduced Amoroso kernel density estimators for non-negative data” (with Kakizawa, Y.), Communications in Statistics-Theory and Methods, Vol.47, Issue 20, pp. 4905-4937, 2018.
“Generalized gamma kernel density estimation for nonnegative data and its bias reduction” (with Kakizawa, Y.), Journal of Nonparametric Statistics, Vol.30, Issue 3, pp. 598-639, 2018.
“Multivariate density estimation using a multivariate weighted log-normal kernel”, Sankhya A, Vol.80, Issue 2, pp.247-266, 2018.
“Inverse gamma kernel density estimation for nonnegative data” (with Kakizawa, Y.), Journal of the Korean Statistical Society, Vol.46, Issue 2, pp. 194-207, 2017.
“Weighted log-normal kernel density estimation”, Communications in Statistics-Theory and Methods, Vol.45, pp. 6670-6687, 2016.
“Bias reductions for beta kernel estimation”, Journal of Nonparametric Statistics, Vol.28, Issue 1, pp.1-30, 2016.
“Bias corrections for some asymmetric kernel estimators” (with Kakizawa, Y.), Journal of Statistical Planning and Inference, Vol.159, pp. 37-63, 2015.
“On improving convergence rate of Bernstein polynomial density estimator” (with Kakizawa, Y.), Journal of Nonparametric Statistics, Vol.26, Issue 1, pp.61-84, 2014.
“Re-formulation of inverse Gaussian, reciprocal inverse Gaussian, and Birnbaum─Saunders kernel estimators” (with Kakizawa, Y.), Statistics and Probability Letters, Vol.84, pp. 235-246, 2014.
学外での活動
所属学会:日本統計学会、日本数学会
最近の研究テーマ
統計学の数理的側面を研究する数理統計学という研究分野で、ノンパラメトリック推定に関する研究を行っています。統計学の推定手法は、標本(データ)が従う確率分布やパラメータ(母数)で表されるモデルをあらかじめ仮定してパラメータを推定するパラメトリック推定と、特定の確率分布やモデルを仮定せずに推定するノンパラメトリック推定に大別できます(中間的なセミパラメトリックという手法もあります)。パラメトリック推定では仮定した確率分布やモデルが正しければノンパラメトリック推定より推定精度は良いのですが、その仮定が間違っていた場合に誤った推定をしてしまう可能性があります。それに対して、ノンパラメトリック推定は、パラメトリック推定ほど強い仮定を置かなくても機能する推定手法です。連続型確率変数の確率分布を確率密度と呼びますが、ノンパラメトリックに確率密度を推定する手法の1つであるカーネル密度推定では、確率密度の台の境界(確率分布の端)で偏り(バイアス)があるという境界問題が知られていて、その境界問題の対処法の1つに非対称カーネル密度推定という手法があります。これまでは、非対称カーネル密度推定について、新たな推定量の提案や推定精度の改善といった研究をしてきました。現在はその非対称カーネル密度推定を応用して、確率密度の比の推定と、確率密度比推定を利用した仮説検定に関する研究を行っています。